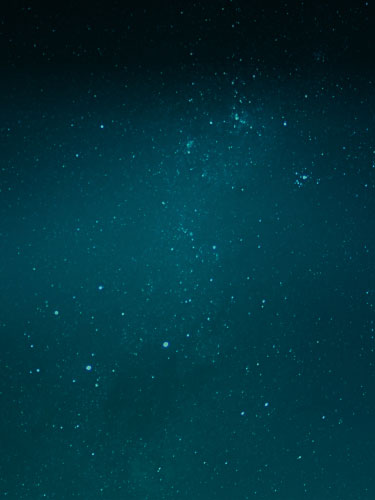
Responsible AI in Privacy & Compliance: How Transparency Builds Trust
Imagine it’s early morning, and you’re preparing for yet another regulatory audit. The regulator has asked detailed questions about your company’s new AI-driven privacy compliance system. You feel confident—but only if you can clearly demonstrate exactly how your AI handles personal data. After all, regulators don’t just expect assurances; they demand evidence.
Privacy professionals working in heavily regulated industries know this feeling all too well. Regulations like GDPR, CCPA, and sector-specific rules in finance or healthcare require proof that your processes for handling personal information are consistent, repeatable, and transparent. But introducing AI often complicates this straightforward equation. AI-driven compliance solutions can appear opaque, raising valid concerns about explainability and trust.
Transparency is essential here—not just as a matter of ethics or good business sense, but as a regulatory imperative. Let’s explore how transparency can help privacy professionals confidently address regulatory scrutiny and build trust in AI systems.
Understanding AI Skepticism in Regulatory Contexts
Privacy professionals are naturally cautious about adopting AI solutions, especially given the potential consequences of privacy breaches or regulatory infractions. High-profile privacy controversies—like Facebook’s Cambridge Analytica scandal or Clearview AI’s facial recognition controversy—demonstrate how quickly trust can erode.
Regulators closely monitor the use of AI tools, demanding clear evidence that personal data is handled appropriately. In highly regulated industries, demonstrating repeatable and explainable privacy practices isn’t just ideal; it’s mandatory. AI systems that cannot transparently justify their decisions risk non-compliance and regulatory fines.
In short, transparency isn’t optional; it’s foundational for regulatory compliance.
Defining Transparency Clearly
Transparency in the context of privacy-focused AI means making the decision-making processes and criteria clear enough for regulators—and internal stakeholders—to easily understand. It doesn’t mean revealing proprietary technology or sensitive business practices. Instead, transparency allows you to clearly document how your AI arrives at specific outcomes when processing personal data.
Privacy professionals must address what’s often called the “Explainability Challenge.”
Regulators want transparency, yet businesses naturally guard proprietary methods. Resolving this tension involves clearly explaining the logic of AI decisions without compromising intellectual property—demonstrating compliance effectively without giving away your competitive advantage.
Transparency Bridges Privacy and Compliance
Privacy teams often emphasize protecting personal data, while compliance teams emphasize documented processes. Transparency links these two priorities effectively. Regulators seek evidence of consistency and repeatability in how companies collect, process, and manage personal data—criteria explicitly required by laws like GDPR’s Accountability Principle.
Transparency provides the evidence regulators require. Clear documentation of AI-driven privacy processes demonstrates to regulators that personal data is managed according to established standards. It turns potentially confusing AI processes into documented, repeatable practices, satisfying regulatory expectations.
Balancing AI and Human Oversight
Automation offers significant advantages in privacy management, reducing manual errors and enhancing consistency. However, regulators still expect human oversight, particularly when nuanced decisions about personal data arise. For example, deciding whether certain data processing activities qualify under legitimate interest or explicit consent often demands human judgment.
Transparency helps reconcile this tension. When AI decision-making processes are clearly documented, demonstrating human oversight becomes straightforward. The combination of automation and transparent human oversight can reassure regulators that your privacy program maintains a high standard of compliance.
Transparency in Action: Real-World Examples
Let’s look at how transparency has influenced compliance outcomes—both positively and negatively:
Positive Examples:
Apple’s Differential Privacy:
- Apple clearly explains its approach to protecting user data through differential privacy. The company openly describes how data collected from user devices is anonymized, aggregated, and protected against re-identification. This transparent explanation reassures regulators and users alike, aligning with GDPR principles.
IBM Watson’s Explainable AI (Healthcare):
- IBM Watson employs explainable AI tools in healthcare settings, providing clear documentation of decision-making processes used to analyze patient data. Regulators and healthcare professionals value these detailed insights, which document precisely how sensitive medical information is handled consistently and responsibly.
Capital One’s Fraud Detection Transparency:
- Capital One openly shares how its AI-driven fraud detection systems analyze transactions, highlighting key factors influencing decisions. This transparency reassures both regulators and customers about responsible use of personal financial information.
Negative Examples:
Amazon Alexa’s Privacy Challenges:
- Amazon faced scrutiny when unclear explanations of how Alexa handled user voice recordings emerged. Ambiguities raised privacy concerns, attracted regulatory attention, and led to fines under GDPR in the EU.
Clearview AI’s Facial Recognition:
- Clearview AI attracted global regulatory action by failing to clearly communicate how user images were sourced, processed, and secured. Lack of transparency and documented consent practices led to regulatory backlash and severe compliance challenges worldwide.
These examples underscore the importance of transparent communication and clear documentation in maintaining regulatory trust and avoiding costly compliance failures.
Practical Tools Supporting Transparency
Several practical tools and frameworks are available to help privacy professionals achieve transparency with AI systems:
IBM AI Explainability 360:
Offers methodologies to document and demonstrate AI decision-making clearly, enabling better regulatory compliance.
Google’s Model Cards:
Clearly document the capabilities and limitations of AI models, assisting regulators and stakeholders in understanding AI-driven decisions without confusion.
NIST AI Risk Management Framework:
Provides comprehensive guidance for managing AI-related risks transparently, supporting regulatory compliance in highly sensitive industries.
Leveraging these tools can significantly streamline the compliance process by ensuring AI-driven practices are well-documented and demonstrable to regulatory bodies.
Communicating Transparency Clearly to Regulators
Effective communication of transparency involves providing regulators clear, concise, and relevant explanations of your AI-driven data handling processes. Transparency documentation should avoid unnecessary technical complexity but remain sufficiently detailed to demonstrate compliance clearly.
Striking this balance requires careful planning. Regulators appreciate documentation that is structured clearly, includes relevant examples, and clearly indicates human oversight where appropriate. Rather than overwhelming regulators with technical information, your explanations should provide clarity on decision-making criteria, oversight, and consistency in privacy practices.
The Future of Transparency in Regulatory Compliance
Looking ahead, transparency will likely become even more critical. With emerging regulations like the European Union’s AI Act, transparency in AI-driven data handling is rapidly transitioning from a competitive differentiator to a baseline regulatory requirement.
Forward-thinking privacy professionals recognize that proactively adopting transparency can simplify compliance management, reduce regulatory risks, and strengthen consumer trust. Companies that transparently demonstrate ethical and compliant AI practices today are positioning themselves favorably for regulatory reviews tomorrow.
Transparency as a Regulatory Asset
For privacy professionals operating in heavily regulated industries, transparency is far more than a concept or a best practice—it’s a regulatory necessity. Clearly documenting and communicating how AI manages personal information satisfies regulatory scrutiny, reduces compliance risks, and enhances stakeholder confidence.
When regulators next examine your privacy compliance processes—and inevitably, they will—transparent documentation will be your strongest asset. Building trust through transparency ensures that your company not only meets but exceeds regulatory expectations, ultimately safeguarding your reputation, your relationships, and your compliance credibility.
Because in privacy compliance, trust isn’t just ideal—it’s essential.